More often than not, the relationship between AI and democracy is depicted as antagonistic. AI is an ‘entity’ rather than a tool – an alternative or even a threat that stands in opposition to human decision-making rather than alongside it.
Yet the practical reality of AI is far more grounded, and potentially far more useful.
Several providers are already adapting AI for use in deliberative democracy and citizens’ participation projects. These applications see AI not as an alternative to human decision making, but a way of facilitating deliberative decision making at previously impossible scales and managing the huge amounts of data involved.
Machine Learning and Natural Language Processing
While not every type or function of AI has yet made inroads with democracy technology, two disciplines in particular are proving themselves useful. Those are machine learning (ML) and natural language processing (NLP).
Machine learning refers to the ability of computer systems to get better at a specific task or set of tasks over time and with the consumption and analysis of more data. For example, an AI might be fed a stream of climate data and progressively get better at predicting weather patterns and developing more accurate models.
There’s an almost poetic connection here to the philosophy underpinning democracy itself. More input means more accurate results – the wisdom of the masses.
Applications in democratic systems and citizen participation might vary from analysing and synthesising citizen contributions to, at some point, synthesising detailed policy and even legislative proposals.
Meanwhile, natural language processing enables computers to understand, and interpret language and text in the same format a person otherwise would. The ‘natural’ component means that far from having to break everything down into code like syntax, we can simply talk or write and the computer understands.
Conversely, natural language processing should also enable that computer to produce language virtually indistinguishable from that which we might produce.
Here, the applications are similarly vast – from the translation software many of us use every day to content creation. Not only is AI capable of writing stories, but translating natural language into visual representations – a trend that has gained some traction in recent months with tools like Dall-E and Midjourney.
As politics is a form of communication, being able to improve and streamline that communication has an enormous impact. Whether that’s in making political messaging more accessible, or facilitating political participation across language barriers.
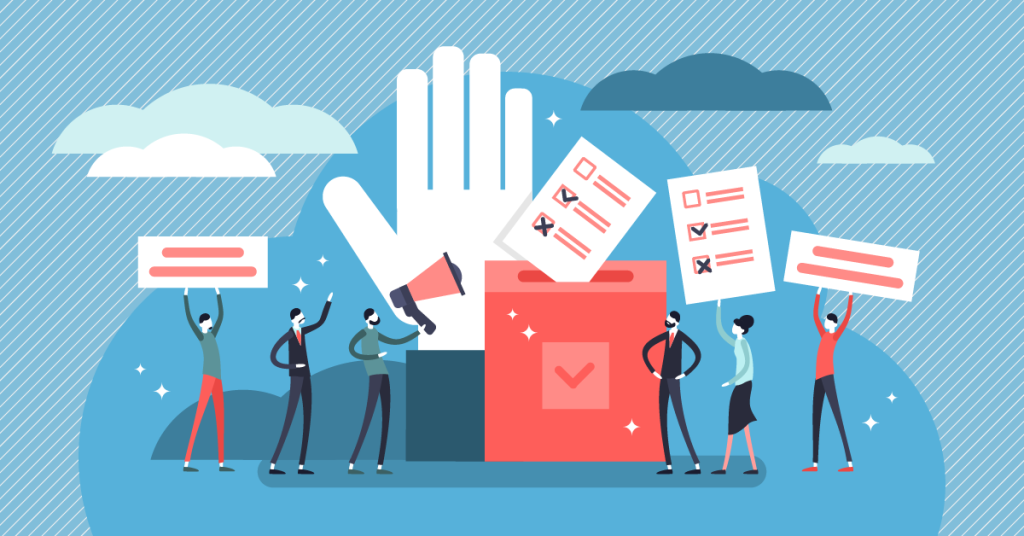
Humans and Machines Working Together
Where these two subfields of AI really come to the fore however is in concert – working together. And perhaps more importantly – working with people.
Digital consultation processes or even simple surveys with the option of open text answers can result in volumes of text that are impossible for public officials to analyse manually. And so, AI supported analysis tools are used to cluster similar answers or even generate summaries.
In 2019 for example, Youth For Climate Belgium used CitizenLab’s NLP technology “to turn thousands of citizen contributions into concise and actionable insights.”
More than 1,700 ideas on how to combat climate change were submitted to an online platform, precipitating more than 2,600 comments and 32,000 votes – a volume that put that CitizenLab’s platform to the test.
Utilising a combination of AI analysis and recommendation with human input to aid in categorisation and the elimination of bias, the result was 15 ‘citizen priorities’ which were then shared back with the community, together with transparent explanations of process.
The project was a learning experience for all – human and AI alike.
CitizenLab’s platform is already in use in the United States, the UK, and beyond. Yet it is not the only tool on the market. Fluicity and Citizens.is are two other tools which, like CitizenLab, generate a visual map of topics that people are talking about based on related keywords they contain.
The person analysing the results is then able to look through the individual entries that were made on a specific topic – a tremendously powerful feature for those dealing with large volumes of information.
The boldest claim made in this regard has come from the makers of CitizenLab, who estimate that it reduces the time needed for the analysis by 90%. Providers also claim that this makes the analysis more objective.
“There is no longer the need for community managers to have a predefined list of categories which removes any potential for bias and focuses on what the community is truly identifying as priorities,” reads the CitizenLab website.
This would seem to stand in contrast to the YouthForClimate example, which as described on CitizenLab’s own blog, called for manual categorisation. However, all of the software providers that we talked to for this article said that it does not replace the analysis by a human – instead augmenting and supporting it, ultimately saving a tremendous amount of time.
The reality would seem to be somewhere in the middle, and when interviewed, this was precisely CitizenLab’s response: “Users can explore our recommendations but they still have the final say if those categorisations are right for them or not. They can approve or reject our suggested topic classifications or they can remove keywords that are not relevant to them.”
In short: AI is a tool for people, not a replacement for them.
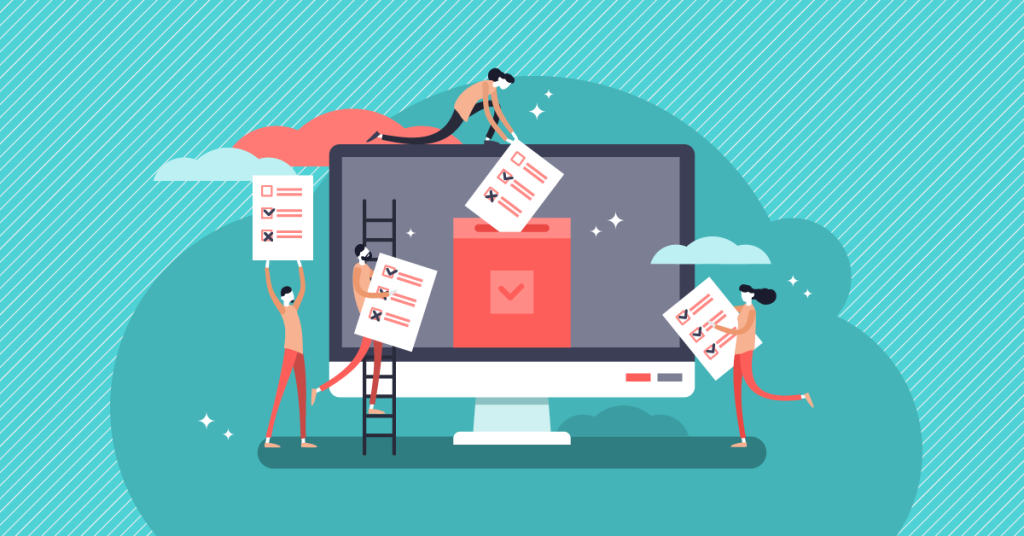
Expanding Possibilities and Challenges
While some of the possibilities with AI tools remain aspirational – such as the ability to assist the writing of policy proposals – many of these existing tools already offer features such as sentiment analysis and toxicity screening.
Sentiment analysis is one of the most common functions of NLP and again works in concert with machine learning. The purpose is to extract key insights from the analysis of volumes of conversation, whether it’s positive, negative or neither. It allows users to understand what people are generally thinking and make decisions accordingly.
Toxicity screening meanwhile is a filtering mechanism which allows the software to automatically screen all entries made by citizens for things like racial slurs or other insults.
While these features might be exceedingly useful in theory, in practice there are questions yet to be answered.
The feedback we have received from civil servants using such tools has been rather mixed. None of them could confirm that it saved them as much time as promised and for some it didn’t save any time at all.
Robert Bjarnason, CEO of the Citizen Foundation, points out that the usefulness of the tools depends on the amount of data involved:
“For any sort of analysis using machine learning and AI to make sense, you actually have to have quite a lot of ideas, quite a lot of comments. If a small municipality does a consultation and they only get 100 ideas, those tools are not really applicable.”
There is also an awareness of the limitations and the importance of doing things right.
“We don’t want to expose the user to a toxicity analysis that is not sort of correct 95% of the time” says Bjarnason, of the decision to withhold toxicity analysis as a feature until it had been fine tuned.
Similarly, CitizenLab points to issues when it comes to accounting for bias – one of the chief concerns of those warning about the impact of AI in governance.
“The model is agnostic to any identifiable citizen attributes but it’s true that human-generated content will carry with it human bias. The AI tools that we are using cannot help us escape from these ingrained biases in society and the tools will just reflect those,” says CitizenLab.
“At this stage, we can only do so much to alleviate the risk of these biases, it mainly has to remain a human attribute to be aware of these risks and correct for them.”
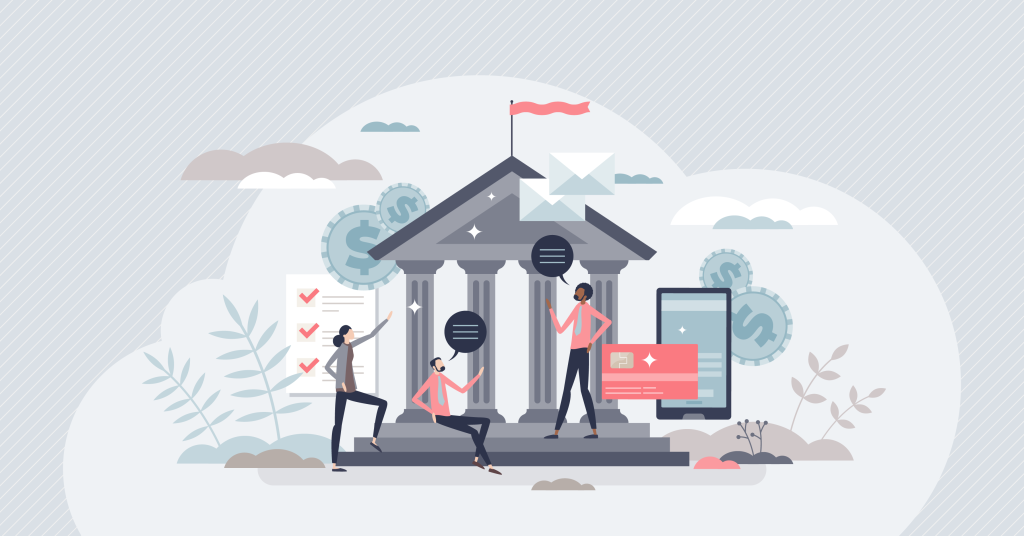
Outlook and Opportunities to Experiment
We already experience the benefits of AI in our daily lives, and regularly come across stories touting the potential such as AI supported cancer detection. However there are also accounts of accidents caused by autonomous cars and racial discrimination by AI based credit scores.
The AI integrated into digital participation tools haven’t yet faced the same degree of public scrutiny, but given that they are meant to be applied to something as fundamental and all-affecting as the democratic process, it is worth taking a closer look.
What we find is that there’s an enormous potential for good and good governance that emerges from our ability to streamline communication and data analysis.
However for now, that potential comes with certain caveats.
First of which is that the effectiveness of certain features scales with the volume of input. The more you feed into it, the more effective the result. Still, this is not dissimilar to democracy itself.
Secondly, bias is an issue. It plagues anything where data input is automated. Yet it is a problem chiefly because it’s a reflection of ourselves.
Thirdly, and perhaps most importantly, it needs people. Depending on your outlook, this can either be a criticism or a saving grace. When it comes to something as important as democracy, keeping a hand on the wheel is deeply important.
As is understanding the risks, challenges and limitations of the technology used. Expecting everyone to build this awareness on their own is probably unrealistic, therefore the AI Ethics Impact Group has made a proposal for an AI ethics label.
Ultimately, the growing number of projects all over the world beginning to use AI in some capacity to inform, facilitate and engage the public in the democratic process present a unique and invaluable opportunity to experiment.